Artificial intelligence: Does this sound a little scary, or does it intrigue you? Never fear, read on to set your mind at ease! We are very excited about how artificial intelligence (AI) is already shaping healthcare, providing faster, more accurate radiologic tests, among many other applications in precision medicine.
In this article, we explore how we have used AI to aid our work in medical education. Prior to incorporating AI, we used audience segmentation based on demographic and interest characteristics. When considering the TRIAD of Essential Elements for AI, Medscape has vast amounts of data that it has been collecting since 1995. Since 2018, Medscape Education began investing in technical expertise in AI and the tools designed to perform sophisticated calculations by employing data scientists with expertise in that medium. In 2019, we began testing these tools; we present the results from our pilot work in chronic obstructive pulmonary disease in this article.
Figure 1. Medscape TRIAD of Essential Elements for AI
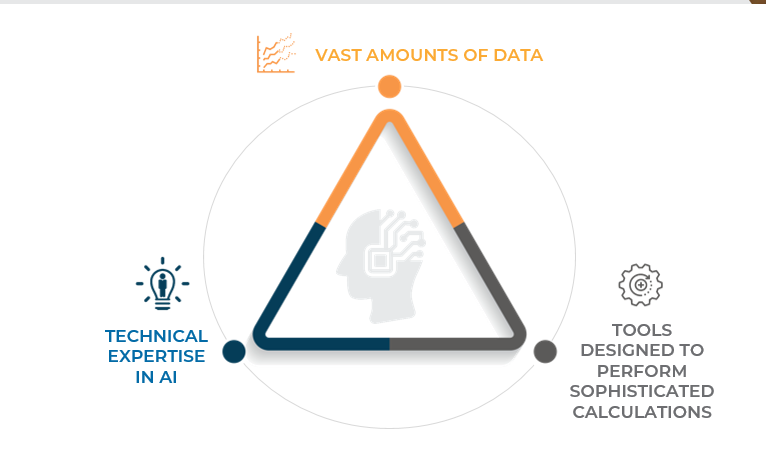
Source: Medscape Education
Our goal in deploying AI in CME was to facilitate increasing the learner’s conversion to “outcomes assessment completer.” Medscape defines “learner” as a member who views content at the web address where the content lives. The “outcomes completer” is a member who completes all outcomes assessment questions (not including the CME post-test, which lives separately from any content and is not used for assessing the education’s impact on desired outcomes).
To improve outcomes assessment completer participation and speed, we employed content recommendations using natural language processing; that is, we searched content and learning objectives for words and phrases that were used in the new program’s content and learning objectives. Then, we generated lists of potential learners based on those who were learners in the matching programs. From those, we narrowed the list to participants who progressed beyond the first page of content and these potential learners received invitations to the new content. We assumed that by targeting learners from similar previous programs and focusing on those who had been engaged deeply with the content, we would also be more likely to increase the proportion of learners who complete the outcomes assessment. This is as opposed to simply recruiting anyone who lists respiratory diseases as an area of interest and practices in the specialty of interest. The results showed a 43% conversion rate for the AI-assisted method compared with the 27% conversion rate for the traditional targeting approach, for an overall increase in conversion percentage from learner to outcomes assessment completer of 59%. This helps us make more precise targeting decisions and may reduce opt-out email rates if we are sending them to a more focused list of members. Interestingly, in this study, the impact of the program on the AI-aided vs traditional method outcomes completers were not significantly different (Figure 2).
Figure 2. Comparison of Percent of Correct Responses for Outcomes Assessment Completers
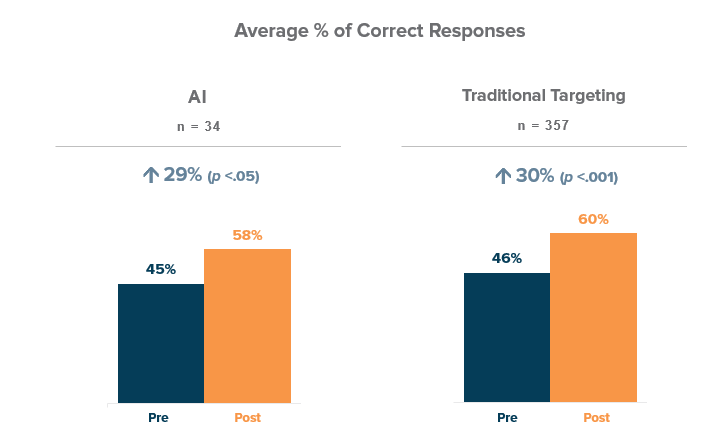
Source: Medscape Chronic Obstructive Pulmonary Disease CME Program (data collected Nov. 15, 2019 to Jan. 7, 2020).
The next step to accompany this approach is to understand which members might benefit most from the education, even if they had not consumed content like it before. To do this, we will identify demographic and information-seeking characteristics of members who have completed a program in a specific content area that they have not in the past to find “look-alike” members who may be considering consuming similar content but have not yet made the leap. This is only one of many exciting ways AI can be used to drive precision education.